Advancements in Soil Behaviour Type (SBT) predictions from Cone Penetration Tests (CPT)
The main objective of this master's thesis is to develop and evaluate SBT predictions from CPT without using laboratory data.
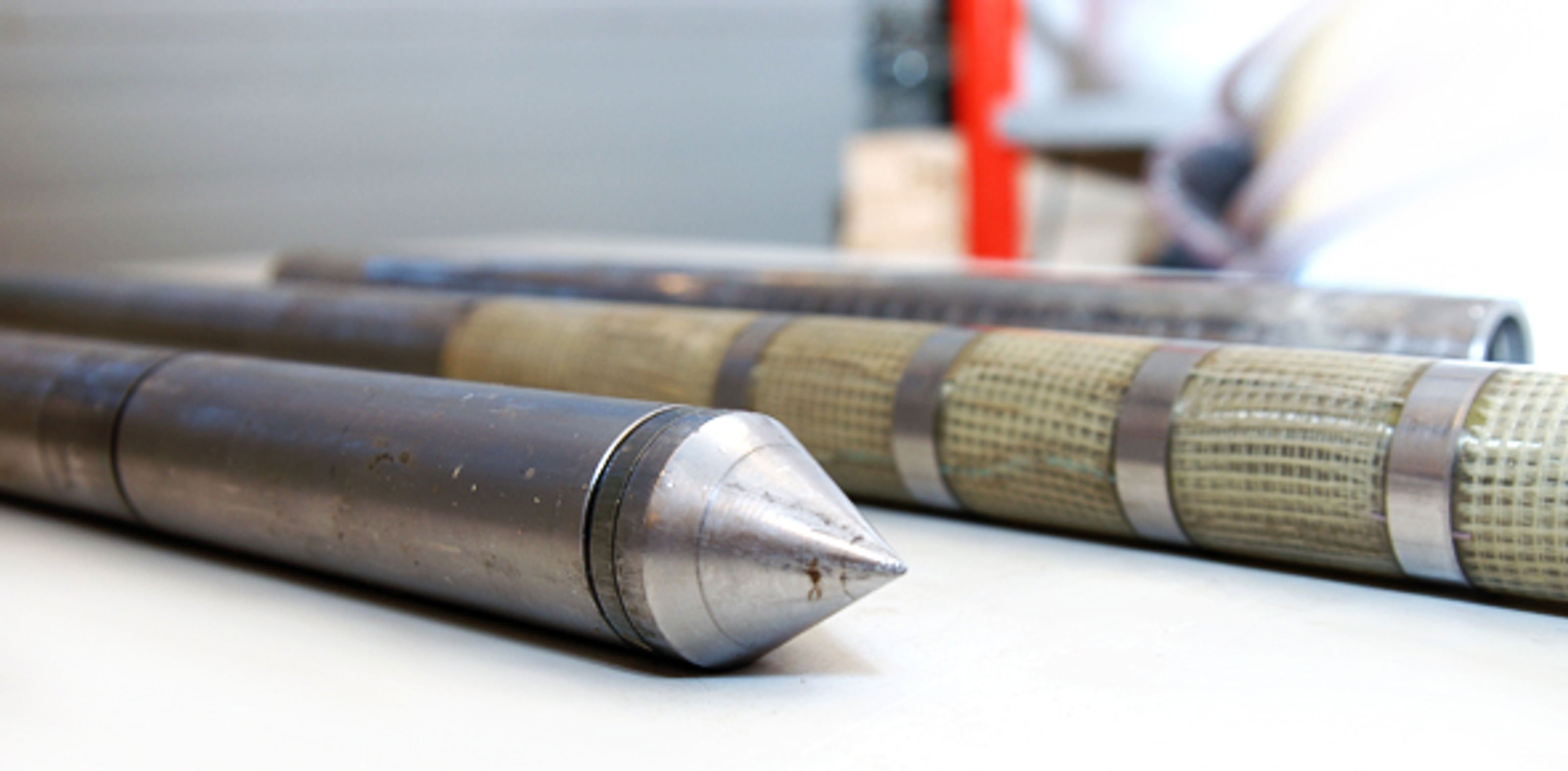
The geotechnical field increasingly embraces digital technologies, significantly improving efficiency, quality, and collaborative efforts. The recently developed Field Manager data platform at NGI is proving to be a central player in this shift. The platform offers a cloud-based repository for storing, viewing, and sharing geotechnical ground survey data.
This project focuses on creating a methodology for automated, early-stage soil classification within Field Manager, utilizing Cone Penetration Test (CPT) data. The CPT is a prevalent in-situ testing method within the geotechnical field, extensively used for deducing various parameters and assessing soil behavior. A notable application of the CPT is determining the Soil Behaviour Type (SBT), which provides insights into the soil composition at each depth measured during the test.
Traditionally, the derivation of SBT relies on empirical correlations found in numerous studies. These correlations typically depend on the in-situ vertical stress, which, in turn, is calculated from the soil unit weight determined through laboratory tests. In the early stages of soil investigation, when laboratory test results are unavailable, it is not uncommon to infer the soil unit weight to assess the Soil Behaviour Type (SBT). Interestingly, some correlations can determine the SBT without needing in-situ vertical stress or laboratory data.
It is worth investigating the accuracy of these correlations, especially in comparison with known sites where both in-situ stresses and soil types have been accurately identified through laboratory tests (e.g., Particle Size Distribution and Atterberg Limits). This assessment might reveal that certain correlations are particularly effective for specific regions in Norway. Furthermore, it may lead to adjustments in the correlations, enhancing their applicability across broader areas.
Depending on the findings, this work may lead to publications and/or be incorporated into Field Manager.
Required background
The student who participates in this project should have a general geoscience background and programming experience in Python.
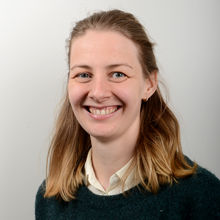
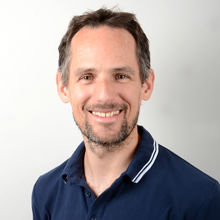